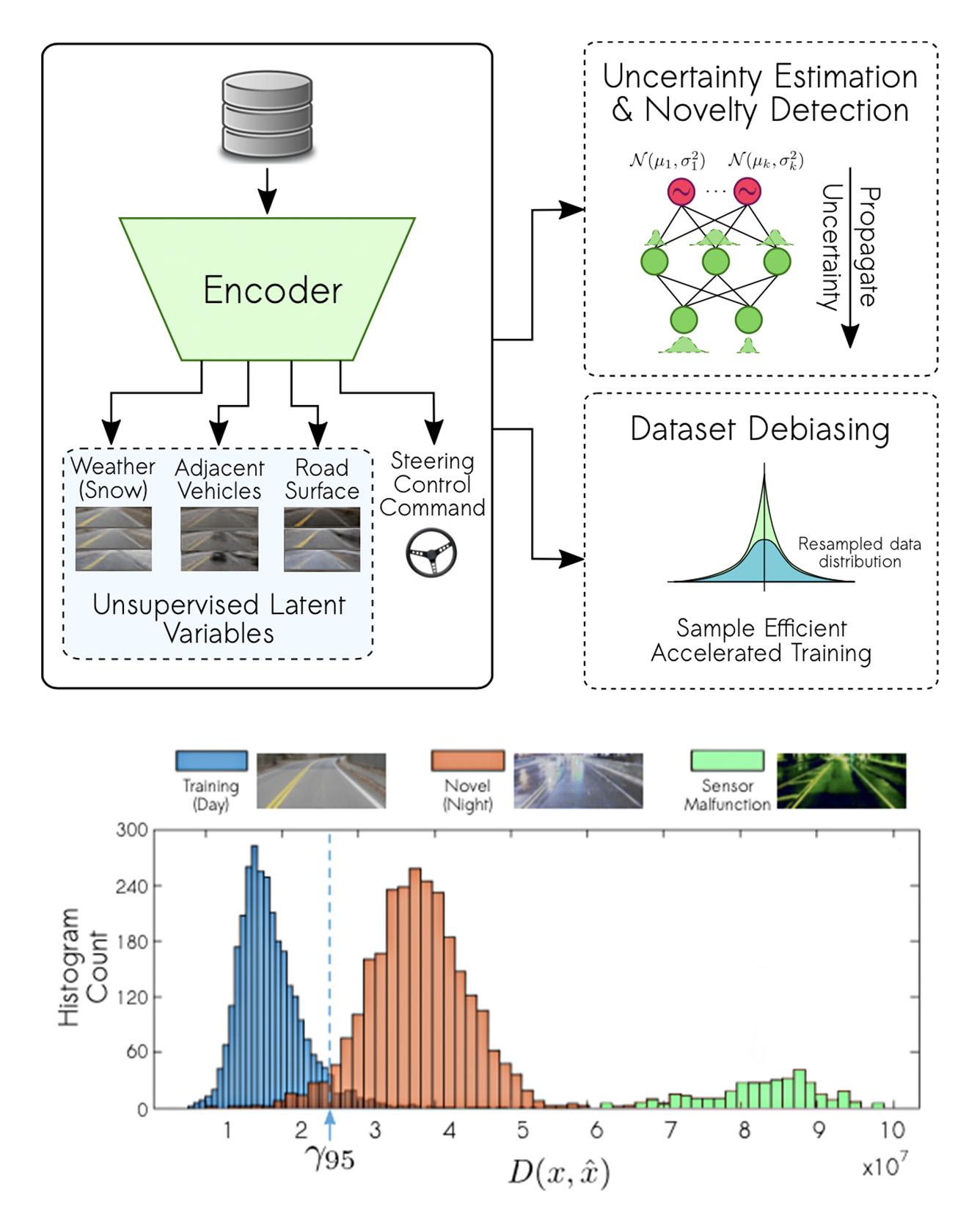
TRI Author: Guy Rosman
All Authors: Alexander Amini, Wilko Schwarting, Guy Rosman, Brandon Araki, Sertac Karaman, Daniela Rus
This paper introduces a new method for end-to-end training of deep neural networks (DNNs) and evaluates it in the context of autonomous driving. DNN training has been shown to result in high accuracy for perception to action learning given sufficient training data. However, the trained models may fail without warning in situations with insufficient or biased training data. In this paper, we propose and evaluate a novel architecture for self-supervised learning of latent variables to detect the insufficiently trained situations. Our method also addresses training data imbalance, by learning a set of underlying latent variables that characterize the training data and evaluate potential biases. We show how these latent distributions can be leveraged to adapt and accelerate the training pipeline by training on only a fraction of the total dataset. We evaluate our approach on a challenging dataset for driving. The data is collected from a full-scale autonomous vehicle. Our method provides qualitative explanation for the latent variables learned in the model. Finally, we show how our model can be additionally trained as an end-to-end controller, directly outputting a steering control command for an autonomous vehicle. Read more
Citation: Amini, Alexander, Wilko Schwarting, Guy Rosman, Brandon Araki, Sertac Karaman, and Daniela Rus. "Variational autoencoder for end-to-end control of autonomous driving with novelty detection and training de-biasing." In 2018 IEEE/RSJ International Conference on Intelligent Robots and Systems (IROS), pp. 568-575. IEEE, 2018.