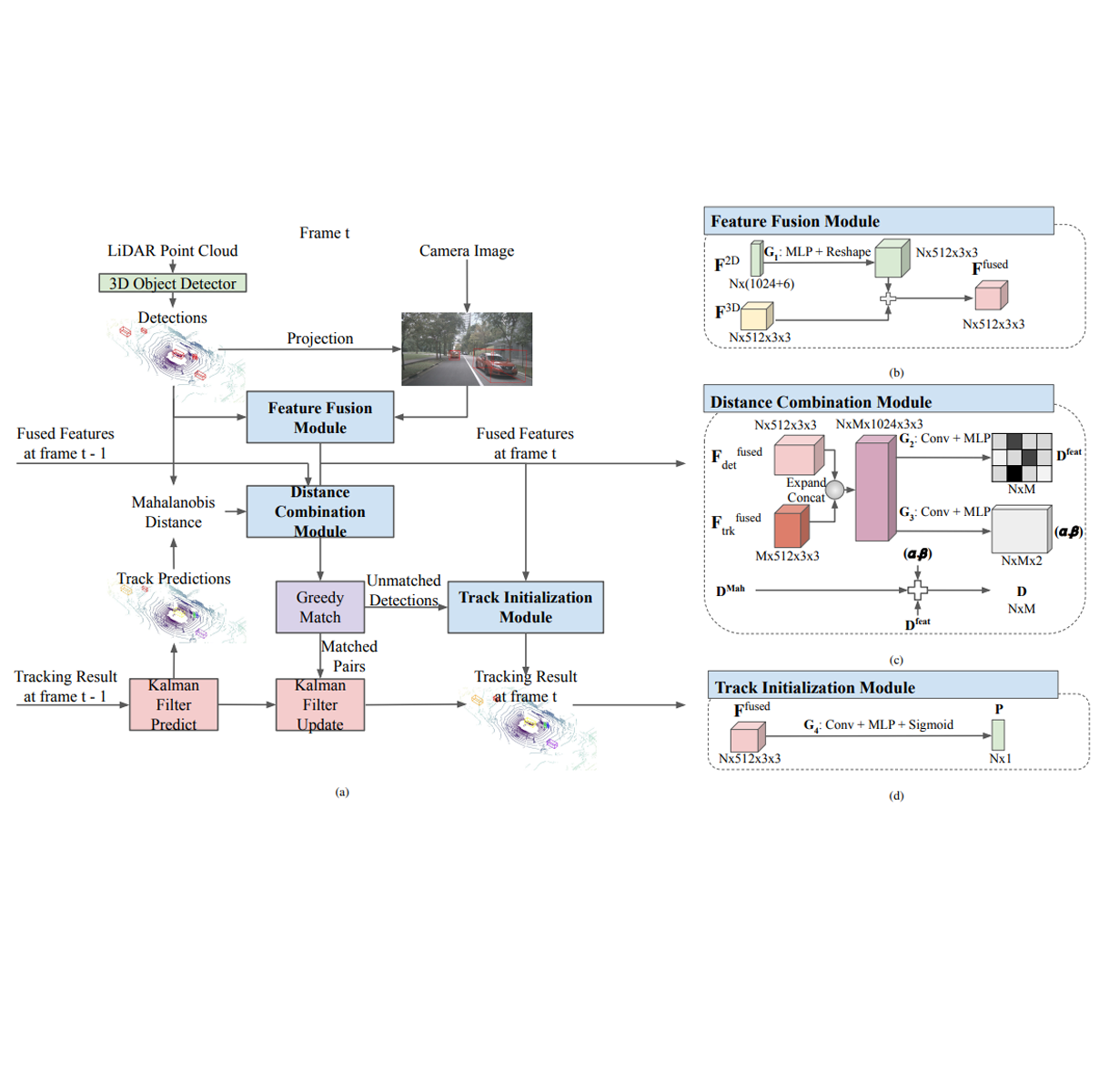
Multi-object tracking is an important ability for an autonomous vehicle to safely navigate a traffic scene. Cur- rent state-of-the-art follows the tracking-by-detection paradigm where existing tracks are associated with detected objects through some distance metric. The key challenges to increase tracking accuracy lie in data association and track life cycle management. We propose a probabilistic, multi-modal, multi- object tracking system consisting of different trainable modules to provide robust and data-driven tracking results. First, we learn how to fuse features from 2D images and 3D LiDAR point clouds to capture the appearance and geometric information of an object. Second, we propose to learn a metric that combines the Mahalanobis and feature distances when comparing a track and a new detection in data association. And third, we propose to learn when to initialize a track from an unmatched object detection. Through extensive quantitative and qualitative results, we show that our method outperforms current state- of-the-art on the NuScenes Tracking dataset. READ MORE
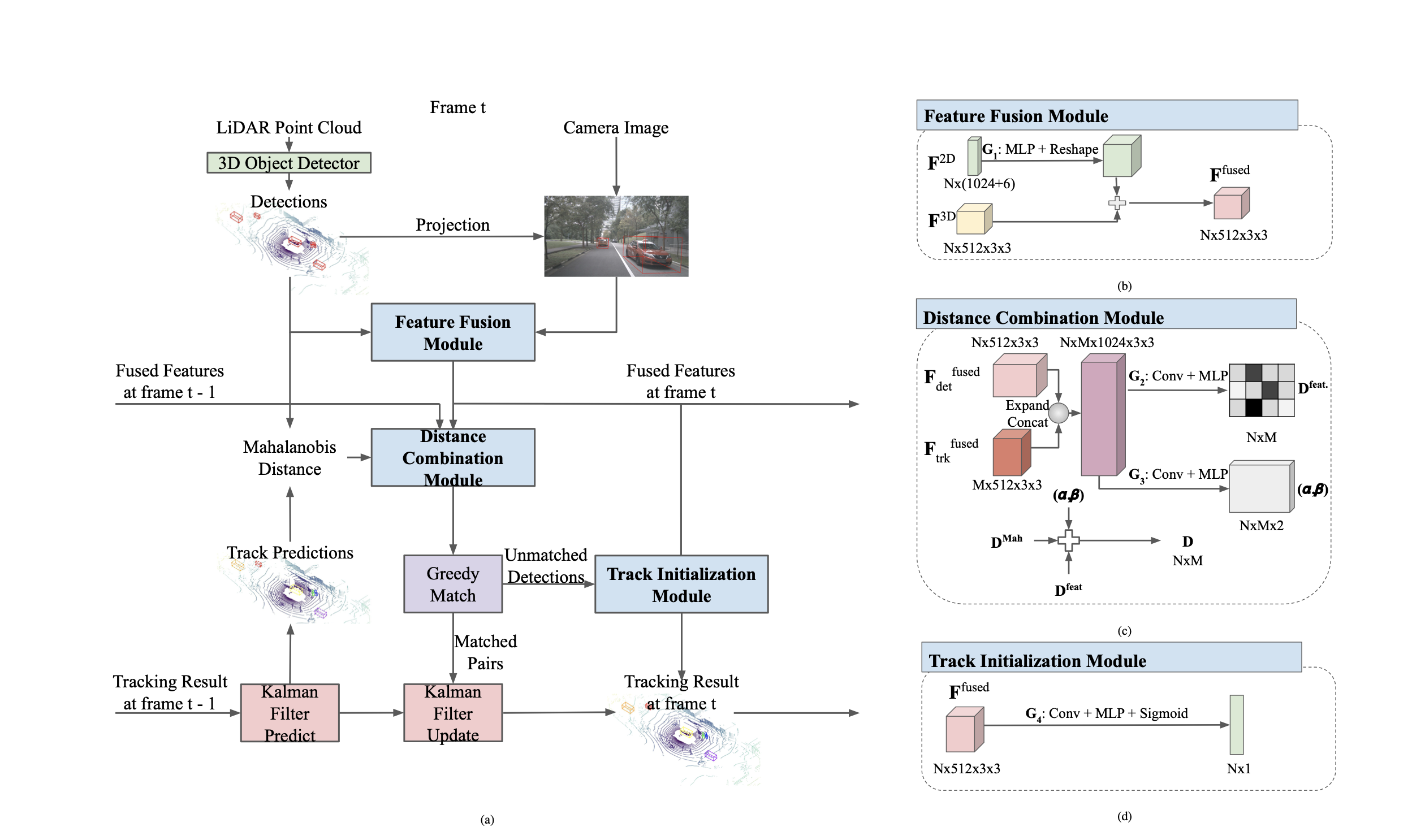