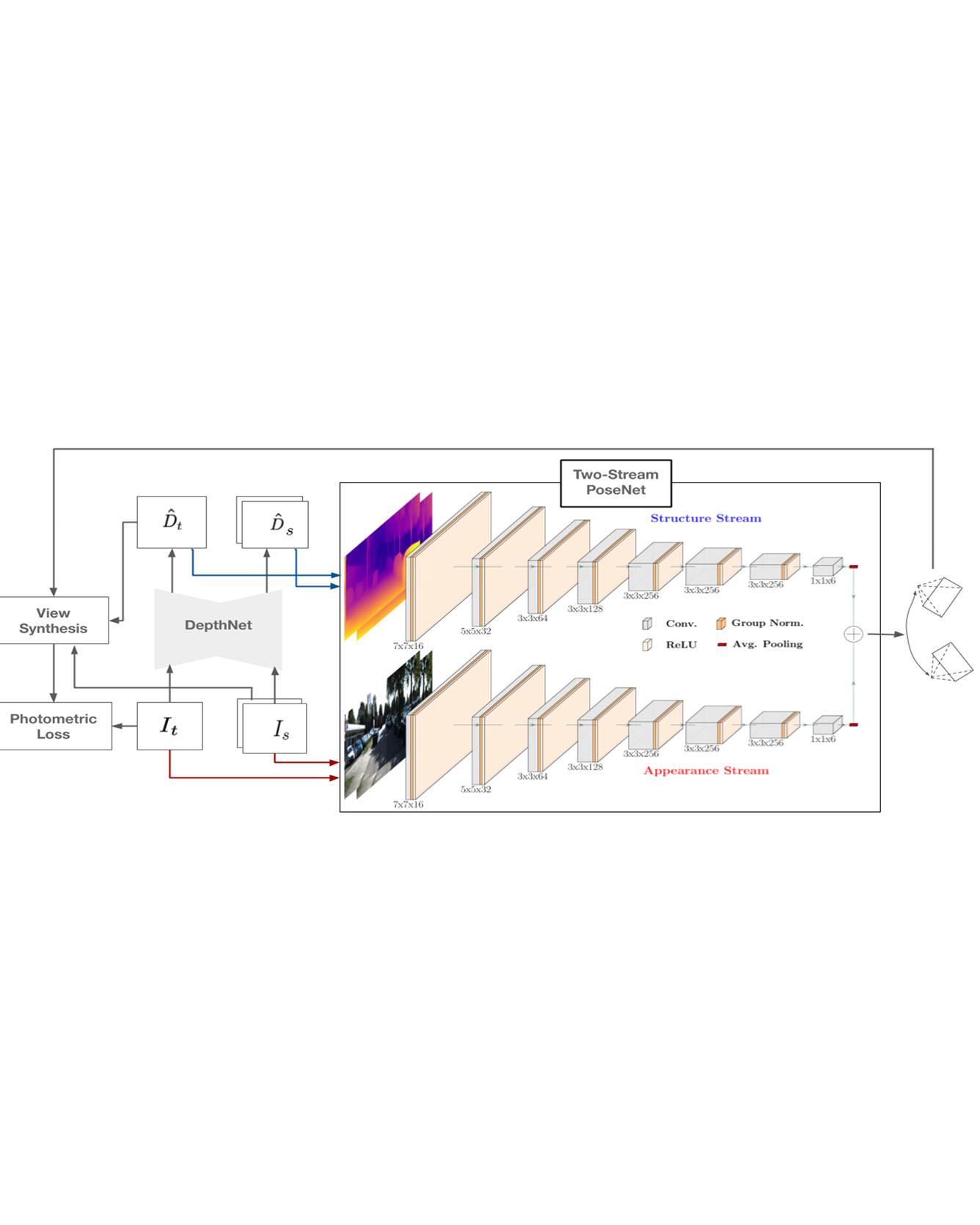
TRI Authors: Rares Ambrus, Vitor Guizilini, Jie Li, Sudeep Pillai, Adrien Gaidon
All Authors: Rares Ambrus, Vitor Guizilini, Jie Li, Sudeep Pillai, Adrien Gaidon
Learning depth and camera ego-motion from raw unlabeled RGB video streams is seeing exciting progress through self-supervision from strong geometric cues. To leverage not only appearance but also scene geometry, we propose a novel self-supervised two-stream network using RGB and inferred depth information for accurate visual odometry. In addition, we introduce a sparsity-inducing data augmentation policy for ego-motion learning that effectively regularizes the pose network to enable stronger generalization performance. As a result, we show that our proposed two-stream pose network achieves state-of-the-art results among learning-based methods on the KITTI odometry benchmark, and is especially suited for self-supervision at scale. Our experiments on a large-scale urban driving dataset of 1 million frames indicate that the performance of our proposed architecture does indeed scale progressively with more data. Read more
Citation: Ambrus, Rares, Vitor Guizilini, Jie Li, Sudeep Pillai, and Adrien Gaidon. "Two stream networks for self-supervised ego-motion estimation." In Conference on Robot Learning (CoRL) 2019.