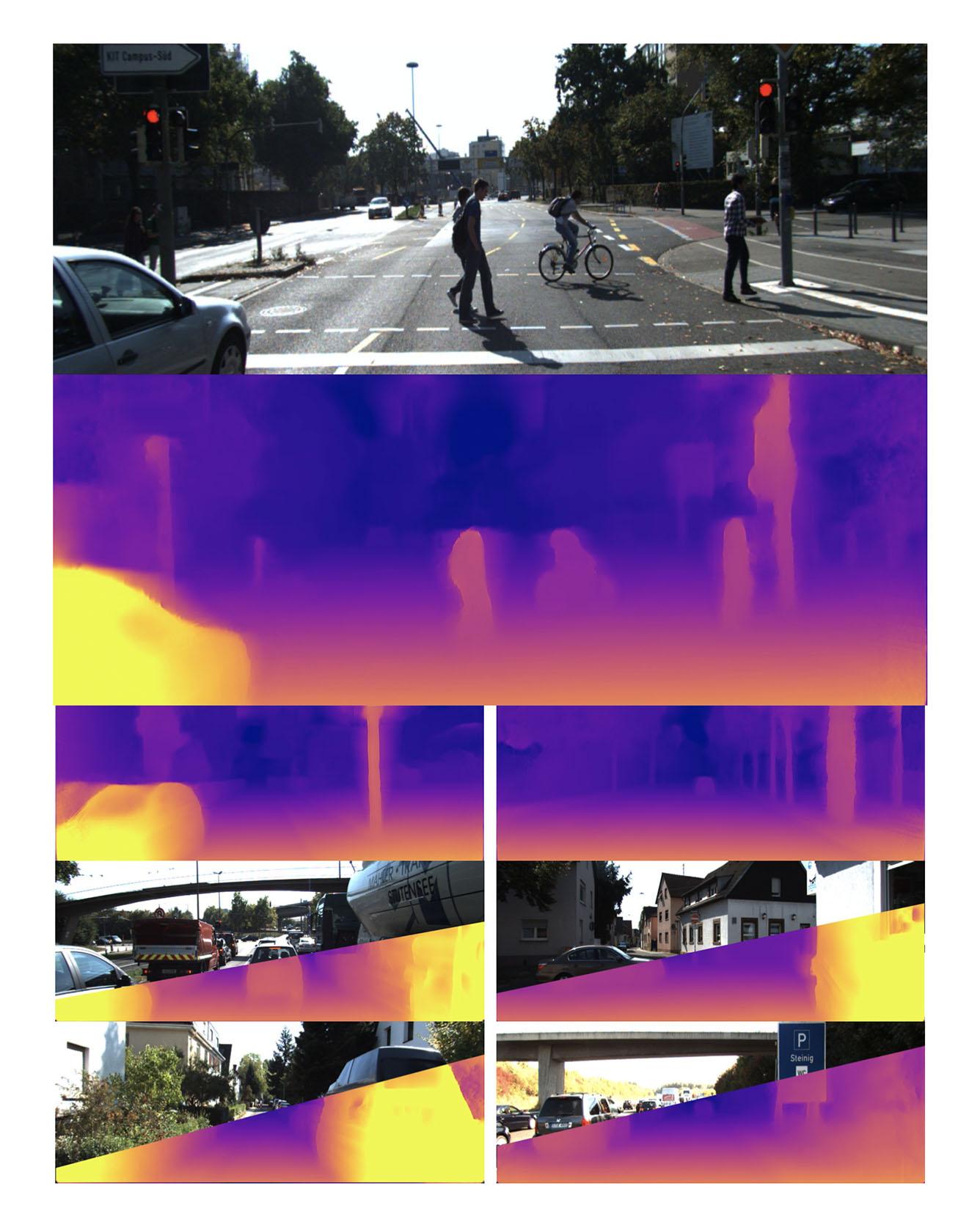
TRI Authors: Sudeep Pillai, Rares Ambrus, Adrien Gaidon
All Authors: Sudeep Pillai, Rares Ambrus, Adrien Gaidon
Recent techniques in self-supervised monocular depth estimation are approaching the performance of supervised methods, but operate in low resolution only. We show that high resolution is key towards high-fidelity self-supervised monocular depth prediction. Inspired by recent deep learning methods for Single-Image Super-Resolution, we propose a sub-pixel convolutional layer extension for depth super-resolution that accurately synthesizes high-resolution disparities from their corresponding low-resolution convolutional features. In addition, we introduce a differentiable flip-augmentation layer that accurately fuses predictions from the image and its horizontally flipped version, reducing the effect of left and right shadow regions generated in the disparity map due to occlusions. Both contributions provide significant performance gains over the state-of-the-art in self-supervised depth and pose estimation on the public KITTI benchmark. A video of our approach can be found at this https URL. Read More
Citation: Pillai, Sudeep, Rareş Ambruş, and Adrien Gaidon. "Superdepth: Self-supervised, super-resolved monocular depth estimation." In 2019 International Conference on Robotics and Automation (ICRA), pp. 9250-9256. IEEE, 2019.