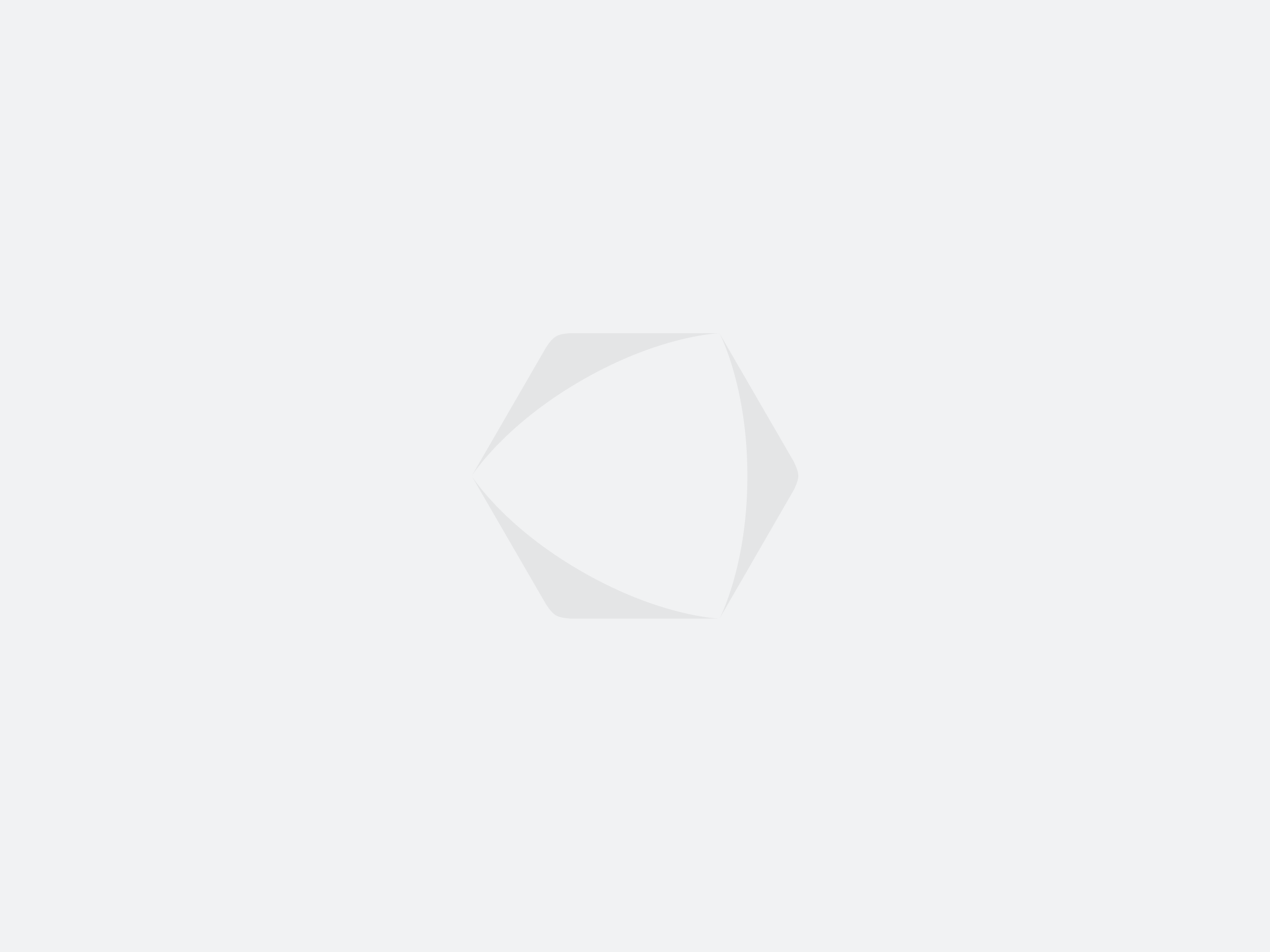
TRI Author: Simon Stent
All Authors: Pablo F. Alcantarilla and Simon Stent and German Ros and Roberto Arroyo and Riccardo Gherardi
We propose a system for performing structural change detection in street-view videos captured by a vehicle-mounted monocular camera over time. Our approach is motivated by the need for more frequent and efficient updates in the large-scale maps used in autonomous vehicle navigation. Our method chains a multi-sensor fusion SLAM and fast dense 3D reconstruction pipeline, which provide coarsely registered image pairs to a deep Deconvolutional Network (DN) for pixel-wise change detection. We investigate two DN architectures for change detection, the first one is based on the idea of stacking contraction and expansion blocks while the second one is based on the idea of Fully Convolutional Networks. To train and evaluate our networks we introduce a new urban change detection dataset which is an order of magnitude larger than existing datasets and contains challenging changes due to seasonal and lighting variations. Our method outperforms existing literature on this dataset, which we make available to the community, and an existing panoramic change detection dataset, demonstrating its wide applicability. Read More
Citation: Alcantarilla, Pablo F., Simon Stent, German Ros, Roberto Arroyo, and Riccardo Gherardi. "Street-view change detection with deconvolutional networks." Autonomous Robots 42, no. 7 (2018): 1301-1322.