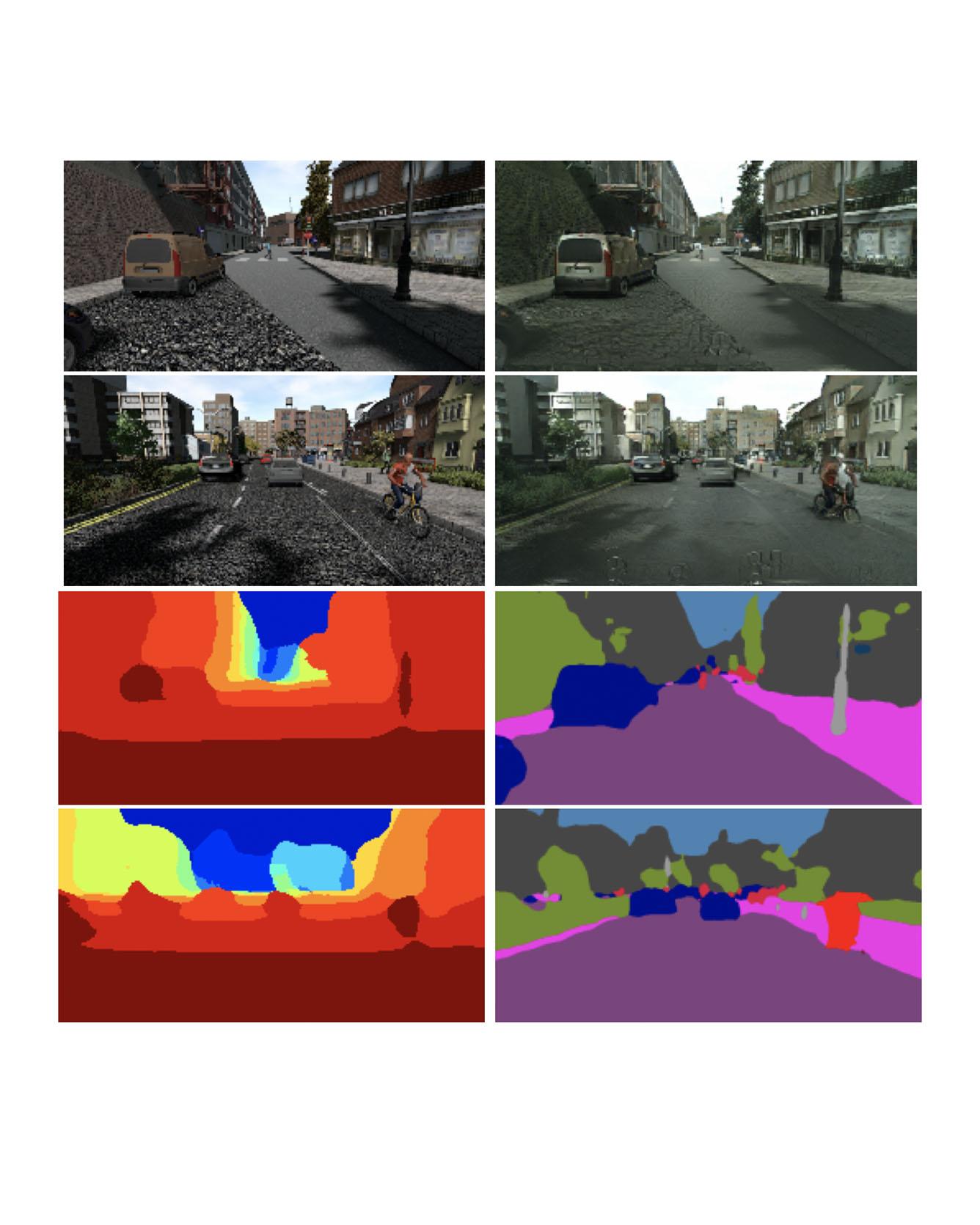
TRI Authors: Kuan-Hui Lee, Jie Li, Adrien Gaidon
All Authors: Kuan-Hui Lee, German Ros, Jie Li, Adrien Gaidon
Deep Learning for Computer Vision depends mainly on the source of supervision.Photo-realistic simulators can generate large-scale automatically labeled syntheticdata, but introduce a domain gap negatively impacting performance. We propose anew unsupervised domain adaptation algorithm, called SPIGAN, relying on Sim-ulator Privileged Information (PI) and Generative Adversarial Networks (GAN).We use internal data from the simulator as PI during the training of a target tasknetwork. We experimentally evaluate our approach on semantic segmentation. Wetrain the networks on real-world Cityscapes and Vistas datasets, using only unla-beled real-world images and synthetic labeled data with z-buffer (depth) PI fromthe SYNTHIA dataset. Our method improves over no adaptation and state-of-the-art unsupervised domain adaptation techniques. Read More
Citation: Lee, Kuan-Hui, German Ros, Jie Li, and Adrien Gaidon. "SPIGAN: Privileged adversarial learning from simulation." In International Conference on Learning Representations, 2019.