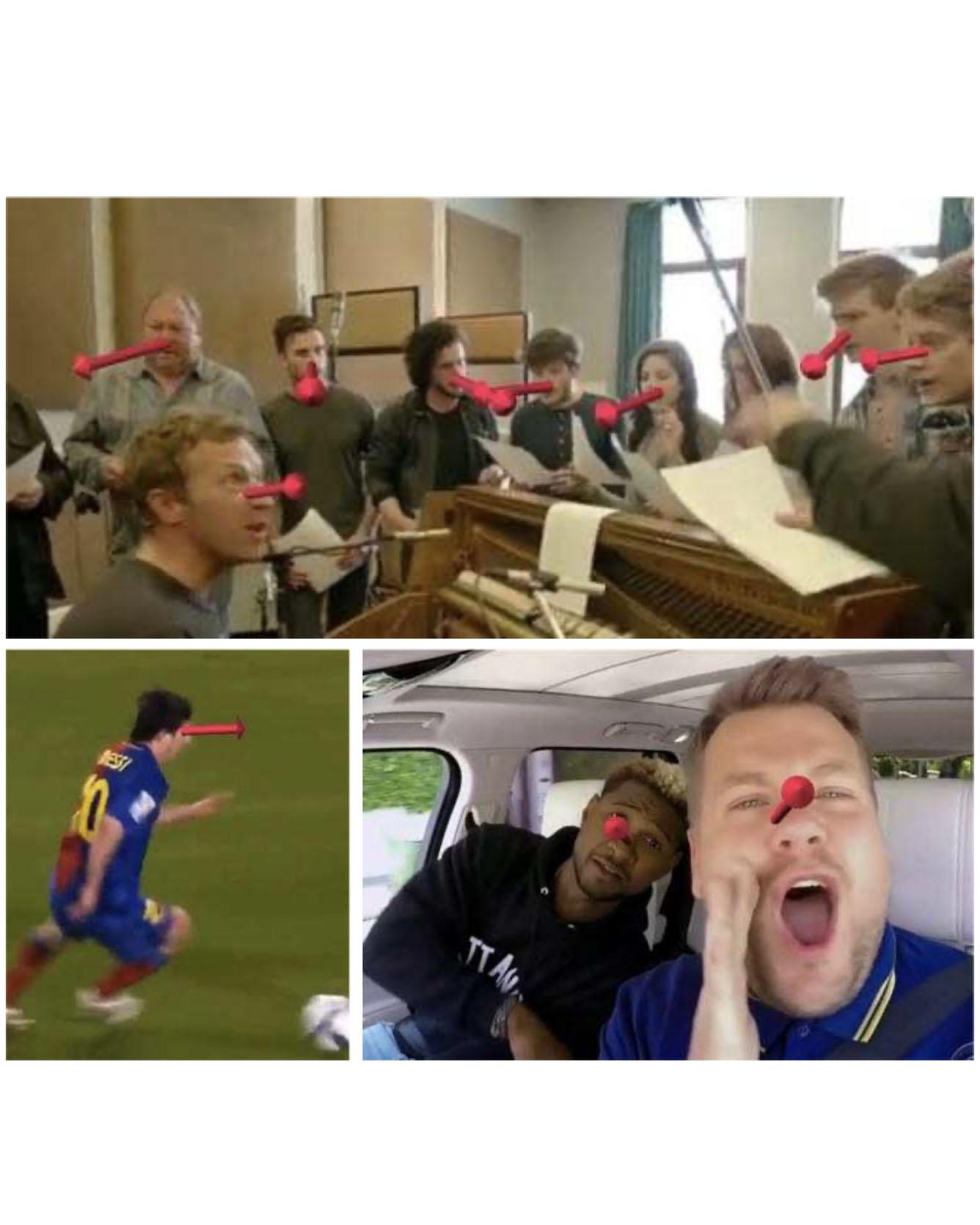
TRI Authors: Simon Stent
All Authors: Petr Kellnhofer, Adrià Recasens, Simon Stent, Wojciech Matusik and Antonio Torralba
Understanding where people are looking is an informative social cue. In this work, we present Gaze360, a large-scale remote gaze-tracking dataset and method for robust 3D gaze estimation in unconstrained images. Our dataset consists of 238 subjects in indoor and outdoor environments with labelled 3D gaze across a wide range of head poses and distances. It is the largest publicly available dataset of its kind by both subject and variety, made possible by a simple and efficient collection method. Our proposed 3D gaze model extends existing models to include temporal information and to directly output an estimate of gaze uncertainty. We demonstrate the benefits of our model via an ablation study, and show its generalization performance via a cross-dataset evaluation against other recent gaze benchmark datasets. We furthermore propose a simple self-supervised approach to improve cross-dataset domain adaptation. Finally, we demonstrate an application of our model for estimating customer attention in a supermarket setting. Our dataset and models will be made available at http://gaze360.csail.mit.edu. Read More
Citation: Kellnhofer, Petr, Adria Recasens, Simon Stent, Wojciech Matusik, and Antonio Torralba. "Gaze360: Physically unconstrained gaze estimation in the wild." In Proceedings of the IEEE International Conference on Computer Vision, pp. 6912-6921. 2019.