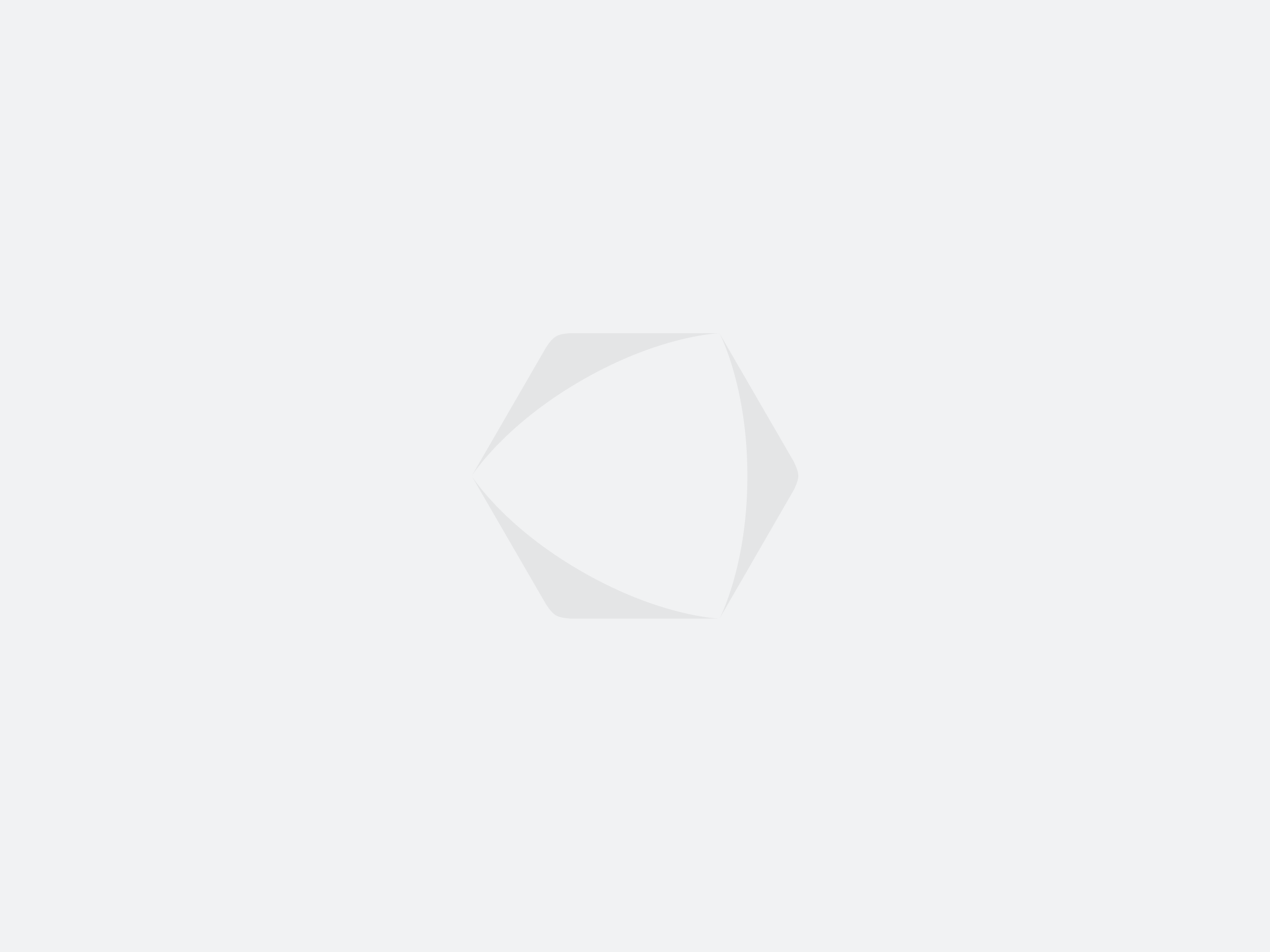
TRI Authors: Kuan-Hui Lee, Yusuke Kanzawa, Matthew Derry
All Authors: Kuan-Hui Lee, Yusuke Kanzawa, Matthew Derry, and Michael R. James
This paper proposes the Permutation Matrix Track Association (PMTA) algorithm to support track-to-track, multi-sensor data fusion for multiple targets in an autonomous driving system. In this system, measurement data from different sensor modalities (LIDAR, radar, and vision) is processed by object trackers operating on each sensor modality independently to create the tracks of the objects. The proposed approach fuses the object track lists from each tracker, first by associating the tracks within each track list, followed by a state estimation (filtering) step. The eventual output is the unified tracks of the objects provided for further autonomous driving processing, such as path and motion planning. The permutation matrix track association (PMTA) algorithm considers both spatial and temporal information to associate object tracks from different sensor modalities. Experimental results show that the proposed approach improves not only the performance of the multipletarget track-to-track fusion, but also stability and robustness in the resulting speed control and decision making in the autonomous driving system. Read More
Citation: Lee, Kuan-Hui, Yusuke Kanzawa, Matthew Derry, and Michael R. James. "Multi-target track-to-track fusion based on permutation matrix track association." In 2018 IEEE Intelligent Vehicles Symposium (IV), pp. 465-470. IEEE, 2018.