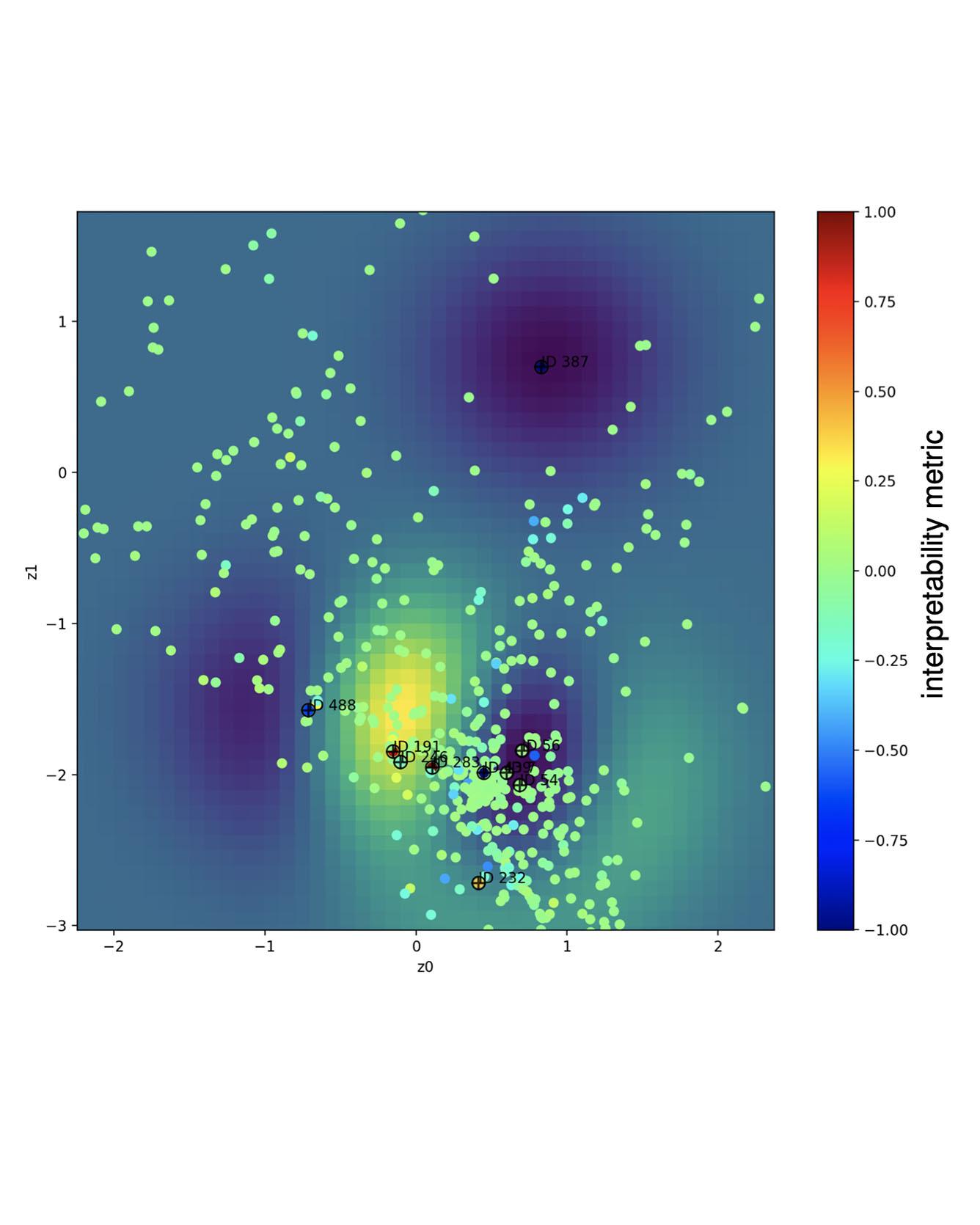
TRI Authors: DeCastro, Jonathan*, Nikos Arechiga
All Authors: DeCastro, Jonathan*, Karen Yan Ming Leung, Nikos Arechiga, Marco Pavone DeCastro, Jonathan*, Karen Yan Ming Leung, Nikos Arechiga, Marco Pavone
We present an approach to interpret parameterized policies through the lens of Signal Temporal Logic (STL). By providing a formally-specified description of desired behaviors we want the policy to produce, we can identify clusters in the parameter space of the policy that can produce the desired behavior. In the context of agent simulation for autonomous driving, this enables an automated way to target and produce challenging scenarios to stress-test the autonomous driving stack and hence accelerate validation and testing. Our approach leverages parametric signal temporal logic (pSTL) formulas to construct an interpretable view on the modeling parameters via a sequence of variational inference problems; one to solve for the pSTL parameters and another to construct a new parameterization satisfying the specification. We perform clustering on the new parameter space using a finite set of examples, either real or simulated, and combine computational graph learning and normalizing flows to form a relationship between these parameters and pSTL formulas either derived by hand or inferred from data. We illustrate the utility of our approach to model selection for validation of the safety properties of an autonomous driving system, using a learned generative model of the surrounding agents. Read More
Citation: DeCastro, Jonathan*, Karen Yan Ming Leung, Nikos Arechiga, Marco Pavone. "Interpretable Policies from Formally-Specified Temporal Properties." 2020 IEEE 23rd International Conference on Intelligent Transportation Systems (ITSC).