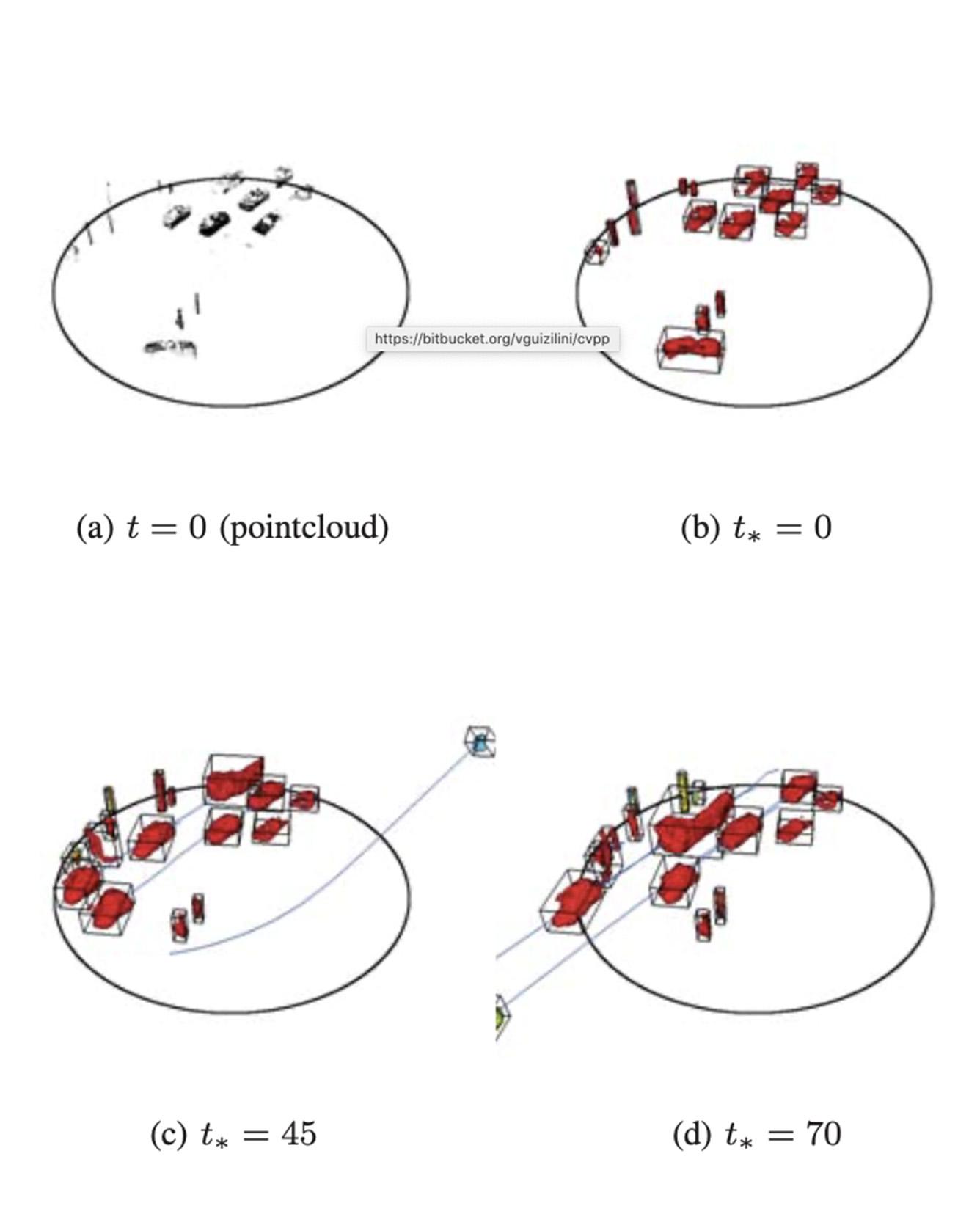
TRI Author: Vitor Guizilini
All Authors: Vitor Guizilini, Ransalu Senanayake, Fabio Ramos
This paper addresses the problem of learning instantaneous occupancy levels of dynamic environments and predicting future occupancy levels. Due to the complexity of most real environments, such as urban streets or crowded areas, the efficient and robust incorporation of temporal dependencies into otherwise static occupancy models remains a challenge. We propose a method to capture the uncertainty of moving objects and incorporate this uncertainty information into a continuous occupancy map represented in a rich high-dimensional feature space. This data-efficient model not only allows us to learn the occupancy states incrementally, but also makes predictions about what the future occupancy states will be. Experiments performed using 2D and 3D laser data collected from crowded unstructured outdoor environments show that the proposed methodology can accurately predict occupancy states for areas of around 1000 m 2 at 10 Hz, making the proposed framework ideal for online applications under real-time constraints. Read More
Citation: Guizilini, Vitor, Ransalu Senanayake, and Fabio Ramos. "Dynamic Hilbert Maps: Real-Time Occupancy Predictions in Changing Environments." In 2019 International Conference on Robotics and Automation (ICRA), pp. 4091-4097. IEEE, 2019.