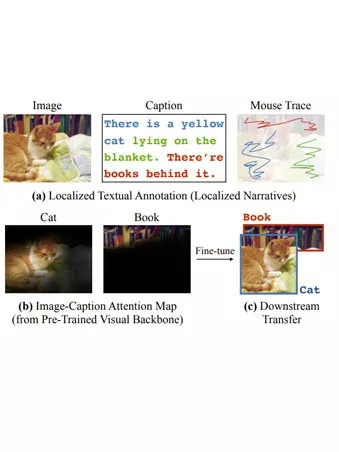
Computer vision tasks such as object detection and semantic/instance segmentation rely on the painstaking annotation of large training datasets. In this paper, we propose LocTex that takes advantage of the low-cost localized textual annotations (i.e., captions and synchronized mouseover gestures) to reduce the annotation effort. We introduce a contrastive pre-training framework between images and captions, and propose to supervise the cross-modal attention map with rendered mouse traces to provide coarse localization signals. Our learned visual features capture rich semantics (from free-form captions) and accurate localization (from mouse traces), which are very effective when transferred to various downstream vision tasks. Compared with ImageNet supervised pre-training, LocTex can reduce the size of the pre-training dataset by 10× or the target dataset by 2× while achieving comparable or even improved performance on COCO instance segmentation. When provided with the same amount of annotations, LocTex achieves around 4% higher accuracy than the previous state-of-the-art “vision+language” pre-training approach on the task of PASCAL VOC image classification. READ MORE