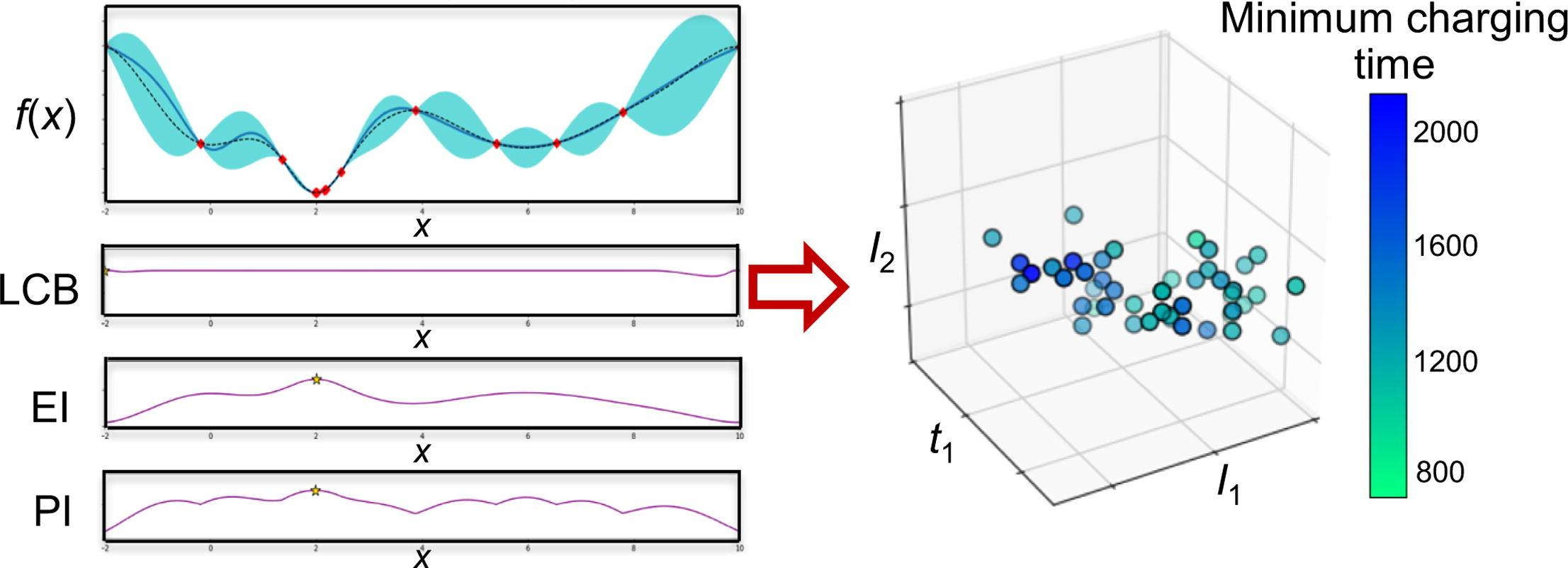
Lithium-ion batteries are one of the most commonly used energy storage device for electric vehicles. As battery chemistries continue to advance, an important question concerns how to efficiently determine charging protocols that best balance the desire for fast charging while limiting battery degradation mechanisms which shorten battery lifetime. Challenges in this optimization are the high dimensionality of the space of possible charging protocols, significant variability between batteries, and limited quantitative information on battery degradation mechanisms. Current approaches to addressing these challenges are model-based optimization and grid search. Optimization based on electrochemical models is limited by uncertainty in the underlying battery degradation mechanisms and grid search methods are expensive in terms of time, testing equipment, and cells. This article proposes a fast-charging Bayesian optimization strategy that explicitly includes constraints that limit degradation. The proposed BO-based charging approaches are sample-efficient and do not require first-principles models. Three different types of acquisition function (i.e., expected improvement, probability of improvement, and lower confidence bound) are evaluated. Their efficacies are compared for exploring and exploiting the parameter space of charging protocols for minimizing the charging time for lithium-ion batteries described by porous electrode theory. The probability-of-improvement acquisition function has lower mean and best minimum charging times than the lower-confidence-bound and expected-improvement acquisition functions. We quantify the decrease in the minimum charging time and increase in its uncertainty with increasing number of current steps used in charging protocols. Understanding ways to increase the convergence rate of Bayesian optimization, and how the convergence scales with the number of degrees of freedom in the optimization, serves as a baseline for extensions of the optimization to include battery design parameters. READ MORE