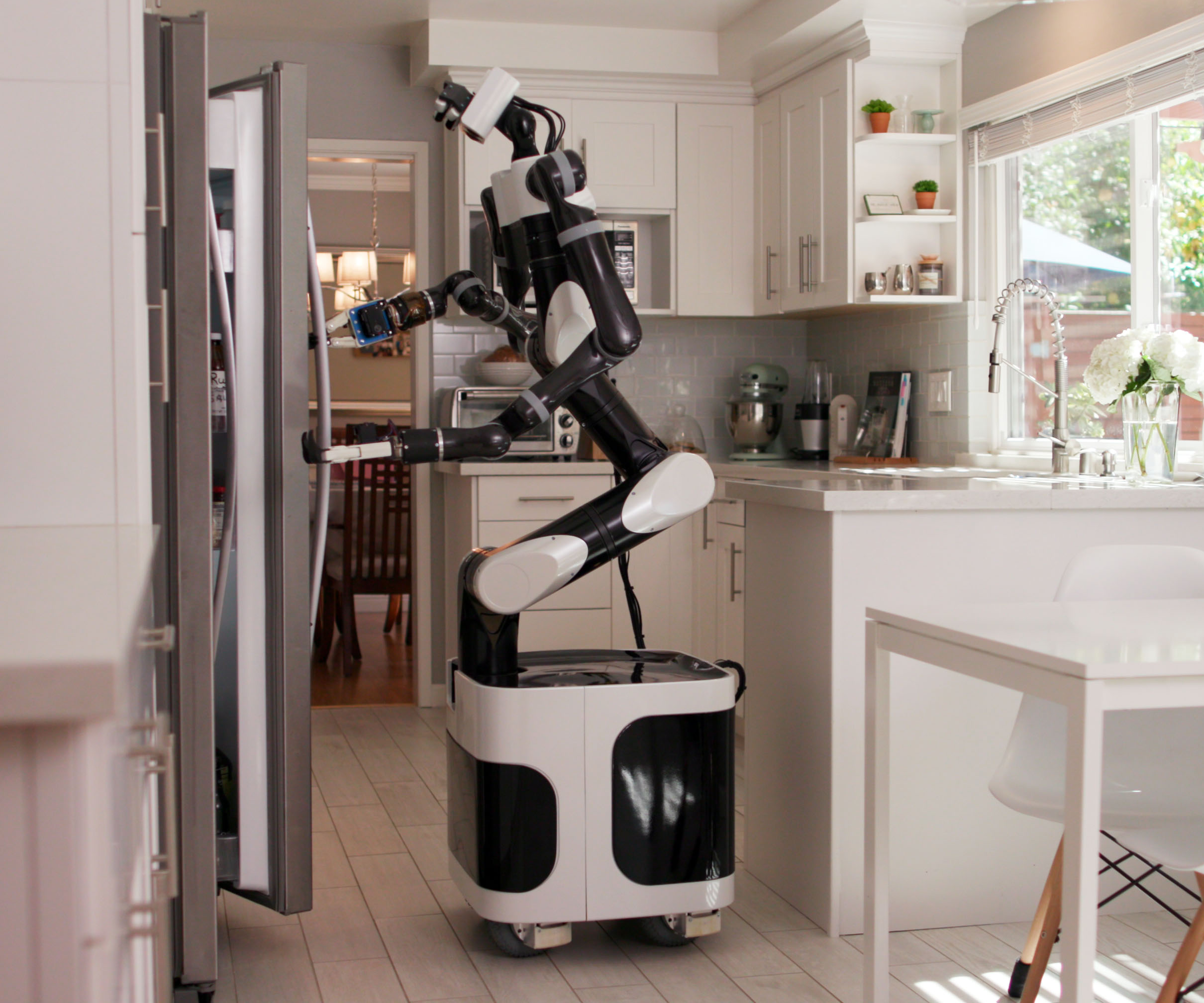
LOS ALTOS, Calif. - The world’s population is aging at a dramatic rate with global implications. Public policy plays a key role in addressing the socioeconomic impact of the demographic shift. But we believe advances in robotic capabilities will be critical to enabling people to age in place longer and live a higher quality life.
The Toyota Research Institute (TRI) is focused on creating and proving the technological breakthroughs necessary to make assistive home robots feasible. In 2015, Gill Pratt, our CEO, professed that the key to the Cambrian explosion of robotics is the combination of cloud robotics and deep learning. This is called fleet learning: if we enable one robot to learn to perform a task, either from a person or in simulation, and then share this knowledge with all other robots, such that they can perform the task in new situations, we can achieve an exponential increase in robotic capabilities.
Earlier this year, Russ Tedrake, TRI’s VP of Robotics Research, posted why simulation is one key aspect for achieving fleet learning and ensuring we can maintain the reliability needed as robots learn. Another is the ability for a person to teach a robot how to perform a task, leveraging human intelligence and insight to guide the robot's physical ability. To help motivate this aspect of fleet learning, we posed a research challenge to teach a general purpose robot to perform useful human-level tasks in real homes. WATCH VIDEO
Operating and navigating in home environments is very challenging for robots. Every home is unique, with a different combination of objects in distinct configurations that change over time. To address the diversity a robot faces in a home environment, we teach the robot to perform arbitrary tasks with a variety of objects, rather than program the robot to perform specific predefined tasks with specific objects. In this way, the robot learns to link what it sees with the actions it is taught. When the robot sees a specific object or scenario again, even if the scene has changed slightly, it knows what actions it can take with respect to what it sees.
We teach the robot using an immersive telepresence system, in which there is a model of the robot, mirroring what the robot is doing. The teacher sees what the robot is seeing live, in 3D, from the robot's sensors. The teacher can select different behaviors to instruct and then annotate the 3D scene, such as associating parts of the scene to a behavior, specifying how to grasp a handle, or drawing the line that defines the axis of rotation of a cabinet door. When teaching a task, a person can try different approaches, making use of their creativity to use the robot's hands and tools to perform the task. This makes leveraging and using different tools easy, allowing humans to quickly transfer their knowledge to the robot for specific situations.
Historically, robots, like most automated cars, continuously perceive their surroundings, predict a safe path, then compute a plan of motions based on this understanding. At the other end of the spectrum, new deep learning methods compute low-level motor actions directly from visual inputs, which requires a significant amount of data from the robot performing the task. We take a middle ground. Our teaching system only needs to understand things around it that are relevant to the behavior being performed. Instead of linking low-level motor actions to what it sees, it uses higher-level behaviors. As a result, our system does not need prior object models or maps. It can be taught to associate a given set of behaviors to arbitrary scenes, objects, and voice commands from a single demonstration of the behavior. This also makes the system easy to understand and makes failure conditions easy to diagnose and reproduce.
Our robot is specifically designed to make teaching and performing these tasks easy. Like a person, it has many redundant degrees of freedom, ensuring the robot can move its hands around in the way that it wants, whenever it wants, by adjusting its whole body posture to accommodate the motions. The robot also has a set of visual and depth cameras with a very wide field of view. This provides a significant amount of context to both the person teaching the robot, and the robot itself.
Our teaching and testing occurs in actual homes. This is critical to achieving sufficient capability and reliability. Our robots are research prototypes, and we select tasks for the robot that motivate and advance algorithm development, rather than demonstrate product concepts. With knowledge gained from our experiments, we constantly iterate and adjust how we are approaching the problems, both in hardware and software. Right now, our system can successfully perform a relatively complex human-level task about 85% of the time. This includes letting the robot automatically try again if it recognizes that it has failed at a specific behavior. Each task is made up of about 45 independent behaviors, which means that every individual behavior results in success, or recoverable failure 99.6% of the time.
Our approach could easily extend beyond homes and be applied to other environments. For example, a person could quickly and remotely teach an industrial arm in a factory to perform repetitive manufacturing tasks, or rapidly adjust a pick-move-pack task for a logistics robot. A key limitation of our approach is that taught tasks cannot currently generalize to other robots or different situations. However, we believe teaching a robot tasks is a promising first step to achieving our broader vision of Fleet Learning, specifically for assisting and empowering people in their home. And we hope that sharing the progress we have made benefits others throughout the robotics community. The technical details of our system are described in depth in a preprint of a publication.
TRI Robotics is looking for passionate and talented team players to join us on our mission. Our open positions are listed on the TRI Robotics Careers webpage.